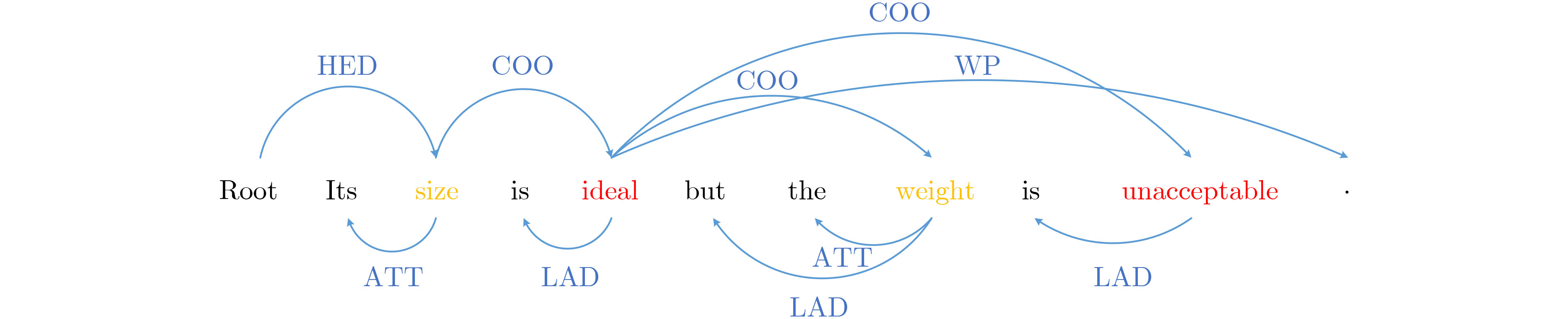
Citation: | LI Bin, CUI Zongyong, WANG Haohan, ZHOU Zheng, TIAN Yu, CAO Zongjie. Exemplar Selection Based on Maximizing Non-overlapping Volume in SAR Target Incremental Recognition[J]. Journal of Electronics & Information Technology, 2024, 46(10): 3918-3927. doi: 10.11999/JEIT240217 |
方面级情感分析,也称细粒度情感分析,是情感分析的一个子领域,旨在识别在线评论中明确给出的方面的情感极性[1]。例如:“The restaurant environment is very good, but the waiter attitude is so bad.”在这句话中,“restaurant environment”和“waiter attitude”是两个不同的方面,可以看出,“restaurant environment”的情感极性是积极的,而“waiter attitude”的情感极性是消极的。由于两个方面的情感极性是截然相反的,因此不能仅考虑句子层面的情感极性。
早期,情感分析问题主要采用知识工程与传统机器学习相结合的方法[2-4]。近年来,以神经网络为代表的深度学习在各个领域取得了显著进展[5-9],由于其可以自动提取特征而不需要人工干预,一些学者采用神经网络解决情感分析问题[7-9]。然而,基于神经网络的方法不能有效地区分句子中每个单词的重要程度,而且句子的情感极性不仅由内容决定,还与所涉及的方面密切相关。为此,一些学者将注意力机制引入情感分类任务中[10-14],以重点关注句子中的方面。文献[10]提出了一种基于注意力机制的长短期记忆网络模型(ATtention-based Long Short-Term Memory network with Aspect Embedding, ATAE-LSTM),当不同的方面作为输入时,注意力机制可以关注句子中的不同部分。文献[11]将记忆网络(Memory Network, MemNet)引入情感分类任务中,并结合多层注意力机制生成方面与上下文的特征表示。文献[12]认为目标和上下文都需要特殊对待,需要使用交互注意力机制来学习各自的表示,因而提出了交互式注意网络(Interactive Attention Networks, IAN)。文献[13]指出IAN模型中的池化操作会忽略句子与目标之间的词对交互,需要以联合的方式对方面和句子进行建模,为此提出一种新的交互注意力模块(Attention-Over-Attention, AOA)。文献[14]提出了一种方面-上下文交互表示结构,该结构通过使用多头注意力机制来生成方面和上下文的特征表示。
虽然注意力机制与传统神经网络相结合的方法已经取得了一定效果,但是这类方法忽略了句子中方面与上下文词之间的句法依存信息以及位置信息,可能导致注意力机制难以学习到合适的注意力权重。具体来说,现有的注意力机制可能导致给定的方面错误地将句法上不相关的上下文词识别为情感词。为了解决这一问题,一些学者将图神经网络(Graph Neural Network, GNN)引入情感分类任务中[15,16],使得句法依存信息得到了有效的利用。文献[15]使用图卷积网络(Graph Convolutional Network, GCN)从句子的依赖树中学习节点表示,并结合注意力机制将其用于情感分类任务。文献[16]提出了一种基于GCN的方面级情感分类模型,该模型可以有效地捕捉句子中多个方面之间的情感依赖关系。此外,方面与上下文词之间的位置信息对识别方面的情感极性来说也是至关重要的,句子中与方面相邻的词要比其他距离较远的词受到更多的关注。为了合理地利用位置信息,文献[17]提出了一种位置感知双向注意力网络模型(Position-aware Bidirectional Attention Network, PBAN),该模型不仅关注方面的位置信息,还利用双向注意力机制相互建模方面和句子之间的关系。文献[18]提出一种基于依存树及距离注意力的句子属性情感分类模型(Dependency Tree and Distance Attention, DTDA),该模型通过计算句子中方面与上下文词的相对距离与语法距离,构造包含语义信息和距离信息的句子特征表示。需要注意的是,上述方法大多对句法依存信息以及位置信息进行单独建模,依存信息以及位置信息并没有得到有效的利用,这使得注意力机制的作用没有得到充分的发挥。为此,本文提出一种面向方面级情感分析的交互图注意力网络模型(an Interactive Graph ATtention networks, IGATs)。本文的贡献如下:
(1)本文对句子中方面与上下文词之间的句法依存信息与位置信息进行了有效的建模,使得模型不仅能够学习到位置信息,还能够捕获到词与词之间的句法依存关系;
(2)模型使用交互注意力机制建模方面与上下文词之间的语义关系,并分别生成方面与上下文的表示,以判断方面的情感极性;
(3)本文在3个公开数据集上评估模型的性能,实验结果表明,该模型对不同数据集的准确率(Accuracy, Acc)与宏平均F1值(Macro average F1, MF1)均有所提升,并进一步证明了句法依存信息与位置信息对模型的重要性。
本文借助Spacy文本处理库中的依存解析器生成句子的句法依存树。以“Its size is ideal but the weight is unacceptable.”为例,其依存树结构如图1所示。其中,黄色字体表示方面词,红色字体表示情感词,有向边表示依存关系,标签表示依存关系类型。为了使句法结构的作用得以充分发挥,本文使用图注意力网络(Graph Attention Network, GAT)编码句法依存树,以捕获词与词之间的句法依存关系。
图注意力网络(GAT)[19]是GNN的一种变体,它通过使用多头注意力机制聚集邻居节点的表示来迭代更新每个节点的表示,实现了对不同邻居节点权重的自适应分配。给定一个有
hl+1i=‖Kk=1σ(∑j∈N(i)alkijWlkhlj) |
(1) |
alkij=exp(LeakyReLU(aTlk[Wlkhli∥Wlkhlj]))∑u∈N(i)exp(LeakyReLU(aTlk[Wlkhli∥Wlkhlu])) |
(2) |
其中,
IGATs模型(如图2)主要由词嵌入层与LSTM层、位置嵌入层、GAT层、交互注意力层与输出层5部分组成。模型的组件在本节其余部分单独介绍。
给定一个包含
位置嵌入层(Position Embedding, PE)采用方面与上下文词之间的距离来衡量二者的相关性,距离分为语法距离和相对距离。语法距离可由句法依存树得到,设方面词
si={1−di2dmax,1≤i<a+1,a+m<i≤n0,a+1≤i≤a+m |
(3) |
通过计算式(3)可以得到句子中方面与上下文词之间的语法距离特征向量
ri={1−a+1−in,1≤i<a+10,a+1≤i≤a+m1−i−a−mn,a+m<i≤n |
(4) |
通过计算式(4)可以得到句子中方面与上下文词之间的相对距离特征向量
pi=ri+si2 |
(5) |
其中,
hi=pihi |
(6) |
本文使用GAT捕获句子中方面与上下文词之间的句法依存关系,GAT层的初始输入为位置嵌入层的输出,为了使位置嵌入层的作用得以充分发挥,本文对每层GATs的输入都进行了位置嵌入,由前文可知,
交互注意力层(Interactive Attention layer, IAtt)使用交互注意力机制建模方面与上下文之间的语义关系,并分别生成方面和上下文的表示。由于GAT层采用多头注意力机制来更新节点的隐藏状态,拼接操作会导致模型参数过多,为了防止过拟合,本文首先引入线性层对GAT层的输出进行降维
hi=Whli+b |
(7) |
其中,
βt=n∑i=1aTihci |
(8) |
αt=exp(βt)n∑i=1exp(βi) |
(9) |
c=n∑t=1αthct |
(10) |
通过计算方面的隐藏状态向量
ϕt=a+m∑i=a+1cTihai |
(11) |
φt=exp(ϕt)a+m∑i=a+1exp(ϕi) |
(12) |
a=a+m∑t=a+1φthat |
(13) |
最后将生成的方面表示
本文将获得的最终表示
p = softmax(Wpr + bp) |
(14) |
其中,
loss=−dp∑i=1yilgpi+λ‖θ‖2 |
(15) |
其中,
为了验证IGATs模型的有效性,本文在3个公开的数据集上进行了实验,分别是Twitter数据集[7]、Laptop数据集 [1]、Restaurant数据集 [1]。这些数据集包含3个标签(即
数据集 | 积极 | 中性 | 消极 |
Twitter-train | 1561 | 3127 | 1560 |
Twitter-test | 173 | 346 | 173 |
Laptop-train | 994 | 464 | 870 |
Laptop-test | 341 | 169 | 128 |
Restaurant-train | 2164 | 637 | 807 |
Restaurant-test | 728 | 196 | 196 |
本文的实验平台如表2所示,实验主要采用Facebook的Pytorch-GPU深度学习框架与Spacy文本处理库,使用python3.7实现。
实验环境 | 具体信息 |
操作系统 | Windows 10 教育版 |
CPU | Intel(R) Core(TM) i7-7700 CPU @ 3.60 GHz |
内存 | 16.0 GB |
显卡 | GTX 1080 |
显存 | 8.0 GB |
本文使用300维的GloVe向量[20]初始化单词嵌入,模型的所有权重参数均采用均匀分布进行初始化。GAT的层数设置为1,图注意力头数设置为1,此时模型的效果最好。模型的超参数设置如表3所示。
超参数 | 超参数值数量 |
词嵌入维度 | 300 |
隐藏状态向量维度 | 300 |
Batch size | 16 |
训练迭代次数epoch | 100 |
优化器Optimizer | Adam |
学习率Learning rate | 0.001 |
Dropout rate | 0.3 |
L2正则化系数 | 0.00001 |
模型采用精准率(Precision, P)、召回率(Recall, R)、准确率(Acc)与宏平均F1值(MF1)等作为评价指标。对于单个类别,设TP为正确预测的样本,
P=TPTP + FN |
(16) |
R=TPTP + FP |
(17) |
F1=2×P×RP+R |
(18) |
对于
Acc=dp∑i=1TPidp∑i=1(TPi+FPi+FNi) |
(19) |
MF1=1dpdp∑i=1F1i |
(20) |
其中,MF1值是指所有类别的F1值的平均,模型重复运行3次,取Acc最高的一次作为最终结果。
为了评估本文的模型,将它与一系列基准模型进行比较,各个模型简介如下所示:
SVM[4]:采用有监督的机器学习方法检测方面术语和方面类别,并检测客户评论中对方面术语和方面类别表达的情感。
LSTM[9]:一种目标相关的长短期记忆(LSTM)模型,该方法在生成句子表示时可以捕捉目标词和其上下文之间的联系,以用于目标相关的情感分类。
MemNet[11]:采用深度记忆网络与多层注意力机制相耦合的方法进行情感分类。
IAN[12]:使用两个注意力网络交互地建模目标与上下文之间的语义关系,并分别生成目标与上下文的表示,为判断方面的情感极性提供了足够的信息。
AOA[13]:对方面和句子进行联合建模,以捕捉方面和上下文之间的相互作用,共同学习方面和句子的表示,并自动地关注句子中的重要部分。
AOA-MultiACIA[14]:一种新的方面-上下文交互表示模型,它能够在其上下文序列建模过程中提取与特定方面相关的特征,并同时生成高质量的方面表示。
ASGCN[15]:通过在句子的句法依存树上构建多层GCN以捕获句法依存信息,并结合注意力机制进行情感分类。
GATs[19]:使用GAT模型进行情感分类,GAT模型描述如2.2节所示。
表4给出了各个模型在3个数据集上的性能对比。可以看出,虽然SVM模型在Laptop和Restaurant数据集上的性能优于LSTM模型,但是它依赖人工特征提取,费时费力且效率低下。基于注意力机制的模型(如MemNet, IAN, AOA, AOA-MultiACIA)性能均优于LSTM模型,这表明注意力机制在情感分析任务中是有效的,而且采用交互注意力机制的IAN与AOA模型性能要优于采用传统注意力机制的MemNet模型,甚至不亚于将句法依存信息考虑在内的ASGCN模型,这表明方面与上下文词都需要受到关注,而且二者之间的交互有利于情感信息的传播,并最终提升情感预测模型的性能。
模型 | Laptop | Restaurant | ||||||
准确率(Acc) | 宏平均F1 | 准确率(Acc) | 宏平均F1 | 准确率(Acc) | 宏平均F1 | |||
SVM | 63.40 | 63.30 | 70.49 | N/A | 80.16 | N/A | ||
LSTM | 69.56 | 67.70 | 69.28 | 63.09 | 78.13 | 67.47 | ||
MemNet | 71.48 | 69.90 | 70.64 | 65.17 | 79.61 | 69.64 | ||
IAN | 72.50 | 70.81 | 72.05 | 67.38 | 79.26 | 70.09 | ||
AOA | 72.30 | 70.20 | 72.62 | 67.52 | 79.97 | 70.42 | ||
AOA-MultiACIA | 72.40 | 69.40 | 75.27 | 70.24 | 82.59 | 72.13 | ||
ASGCN | 72.15 | 70.40 | 75.55 | 71.05 | 80.77 | 72.02 | ||
GATs | 73.12 | 71.25 | 74.61 | 70.51 | 80.63 | 70.41 | ||
IGATs | 75.29 | 73.40 | 76.02 | 72.05 | 82.32 | 73.99 |
然而,上述模型要么只考虑句法依存信息或位置信息,要么只关注于方面与上下文词之间的交互,难以实现二者之间的协调优化。为此,本文提出一个新的模型,即IGATs。可以看出,IGATs在3个数据集上的Acc与MF1值整体上优于其他模型。相较于仅包含多层注意力机制的AOA-MultiACIA模型,IGATs在Twitter数据集上的Acc与MF1分别提升了2.89%和4%,在Laptop数据集上的Acc与MF1分别提升了0.75%和1.81%,在Restaurant数据集上的MF1提升了1.86%,这表明句法依存信息与位置信息有利于识别方面的情感极性,而且Twitter数据集对句法信息与位置信息更加敏感。需要注意的是,IGATs在Restaurant数据集上的Acc稍低于AOA-MultiACIA模型,这是因为该模型采用多层交互注意力机制,它能够在上下文序列建模过程中提取与特定方面相关的特征,并生成高质量的方面表示。相较于将句法依存信息与相对位置信息考虑在内的ASGCN模型,IGATs在Twitter数据集上的Acc与MF1分别提升了3.14%和3%,在Laptop数据集上的Acc与MF1分别提升了0.47%和1%,在Restaurant数据集上的Acc与MF1分别提升了1.55%和1.97%,相较于将句法依存信息与位置信息考虑在内的GATs模型,IGATs在各个数据集上的Acc与MF1也均有所提升,这表明依存信息与位置信息需要结合交互注意力网络才能发挥更好的效果。
除此之外,文章统计了各个模型的可训练参数数量(1M=1Million),如表5所示。由于SVM模型采用人工提取特征的方法进行情感分类,模型性能的好坏很大程度上取决于特征工程,其效率很低。MemNet模型的参数数量最少,其性能虽然优于LSTM,但是仍不及其他模型;IAN模型的参数规模与ASGCN相当,然而其在Laptop与Restaurant上的性能远不及ASGCN;AOA模型借鉴了IAN模型的交互注意力机制,其模型计算复杂度不亚于IAN,但是二者性能很接近;AOA-MultiACIA模型在AOA模型的基础上叠加了多层多头注意力机制,虽然其在各个数据集上的性能不亚于ASGCN,但是模型计算复杂度远高于AOA,更高于ASGCN;GATs模型的参数规模比ASGCN少,二者性能却十分接近,因此,模型效率高于ASGCN模型;IGATs模型的参数规模与GATs接近,但是其性能整体上优于其他模型,这表明IGATs模型的效率远高于其他模型。
模型 | 可训练参数数量 |
SVM | – |
LSTM | 0.72 |
MemNet | 0.36 |
IAN | 2.17 |
AOA | 2.10 |
ASGCN | 2.17 |
GATs | 1.81 |
IGATs | 1.81 |
为了进一步检查IGATs的每个组件对性能的影响,本文对IGATs进行了消融研究,具体如下:
BiLSTM+IAtt:模型由LSTM层与IAtt层组成,LSTM层用来学习句子的语义特征表示,IAtt层用来建立方面与上下文词之间的语义关系。
BiLSTM+GAT+IAtt:模型仅在LSTM层后新增了一个GAT层,用来捕获方面与上下文词之间的句法依存关系。
BiLSTM+PE+IAtt:模型仅在LSTM层后新增了一个PE层,用来将位置信息嵌入到句子的语义特征表示中。
IGATs:完整的模型,不仅考虑了位置信息,还考虑了句法依存信息。
各个模型的性能对比如表6所示。可以看出,BiLSTM+IAtt模型在3个数据集的Acc与MF1值整体上不及其他模型,这表明句子中方面与上下文词之间的句法依存信息与位置信息是不可忽略的。BiLSTM+GAT+IAtt模型的性能整体上优于BiLSTM+IAtt模型,但仍不及IGATs,这表明虽然句法依存信息有利于识别方面的情感极性,但是也不能忽略了位置信息的影响。BiLSTM+PE+IAtt模型的性能在Twitter数据集上比IGATs低,在Laptop数据集上要优于IGATs,在Restaurant数据集上的性能接近IGATs,这表明相较于句法依存信息,Laptop对位置信息更敏感。由此可见,PE层对IGATs模型的贡献最大,其次是GAT层,但是也不能忽视GAT层的影响。
模型 | Laptop | Restaurant | ||||||
准确率(Acc) | 宏平均F1 | 准确率(Acc) | 宏平均F1 | 准确率(Acc) | 宏平均F1 | |||
BiLSTM+IAtt | 74.13 | 72.86 | 75.08 | 70.82 | 81.25 | 72.14 | ||
BiLSTM+GAT+IAtt | 74.86 | 72.98 | 74.92 | 71.08 | 82.05 | 73.45 | ||
BiLSTM+PE+IAtt | 74.42 | 72.35 | 76.65 | 72.75 | 82.23 | 74.01 | ||
IGATs | 75.29 | 73.40 | 76.02 | 72.05 | 82.32 | 73.99 |
由于GAT层包含多层GAT,每层GAT又可能包含多个图注意力头,不能忽视这些因素对模型性能的影响。因此,本文研究了GAT头数
除此之外,本文也研究了GAT层数为1的情况下,图注意力头数K对IGATs最终性能的影响。通过改变K∈{1, 2, 3, 4, 5, 6, 7, 8, 9, 10}的值,测试IGATs在Restaurant数据集上的Acc和MF1值。实验结果如图4所示。可以看出,IGATs的准确率Acc随着K的增加整体呈现下降趋势,MF1值在图注意力头数为6时最大,随后开始下降。综合各方面因素,本文将GAT层数以及图注意力头数均设为1。
本文提出一种面向方面级情感分析的交互图注意力网络模型(IGATs)。首先,通过计算方面与上下文词之间的语法距离与相对距离实现了位置信息的利用;其次,通过构建句法依存树和图注意力网络实现了句法依存信息的融合;最后,通过建立方面与上下文词之间的交互注意力模型,实现了两者之间的协调优化。实验结果表明IGATs能够有效捕获方面与上下文词之间的句法依存信息和位置信息,模型在多个数据集上的评价性能都得到了显著提升。同时,模型仍然存在一些有待研究的内容,例如,没有考虑方面与上下文词之间的依存关系标签以及词性对任务的影响,这些将在今后的工作中进行完善。
[1] |
LI Jianwei, YU Zhentao, YU Lu, et al. A comprehensive survey on SAR ATR in deep-learning era[J]. Remote Sensing, 2023, 15(5): 1454. doi: 10.3390/rs15051454.
|
[2] |
WANG Chengwei, LUO Siyi, PEI Jifang, et al. Crucial feature capture and discrimination for limited training data SAR ATR[J]. ISPRS Journal of Photogrammetry and Remote Sensing, 2023, 204: 291–305. doi: 10.1016/j.isprsjprs.2023.09.014.
|
[3] |
徐丰, 王海鹏, 金亚秋. 深度学习在SAR目标识别与地物分类中的应用[J]. 雷达学报, 2017, 6(2): 136–148. doi: 10.12000/JR16130.
XU Feng, WANG Haipeng, and JIN Yaqiu. Deep learning as applied in SAR target recognition and terrain classification[J]. Journal of Radars, 2017, 6(2): 136–148. doi: 10.12000/JR16130.
|
[4] |
CARUANA R. Multitask learning[J]. Machine Learning, 1997, 28(1): 41–75. doi: 10.1023/A:1007379606734.
|
[5] |
MCCLOSKEY M and COHEN N J. Catastrophic interference in connectionist networks: The sequential learning problem[J]. Psychology of Learning and Motivation, 1989, 24: 109–165. doi: 10.1016/S0079-7421(08)60536-8.
|
[6] |
CHAUDHRY A, DOKANIA P K, AJANTHAN T, et al. Riemannian walk for incremental learning: Understanding forgetting and intransigence[C]. Proceedings of the 15th European Conference on Computer Vision (ECCV), Munich, Germany, 2018: 556–572. doi: 10.1007/978-3-030-01252-6_33.
|
[7] |
DANG Sihang, CAO Zongjie, CUI Zongyong, et al. Class boundary exemplar selection based incremental learning for automatic target recognition[J]. IEEE Transactions on Geoscience and Remote Sensing, 2020, 58(8): 5782–5792. doi: 10.1109/TGRS.2020.2970076.
|
[8] |
MITTAL S, GALESSO S, and BROX T. Essentials for class incremental learning[C]. Proceedings of the IEEE/CVF Conference on Computer Vision and Pattern Recognition, Nashville, USA, 2021: 3528–3517. doi: 10.1109/CVPRW53098.2021.00390.
|
[9] |
REBUFFI S A, KOLESNIKOV A, SPERL G, et al. iCaRL: Incremental classifier and representation learning[C]. Proceedings of the IEEE Conference on Computer Vision and Pattern Recognition, Honolulu, USA, 2017: 5533–5542. doi: 10.1109/CVPR.2017.587.
|
[10] |
DE LANGE M, ALJUNDI R, MASANA M, et al. A continual learning survey: Defying forgetting in classification tasks[J]. IEEE Transactions on Pattern Analysis and Machine Intelligence, 2022, 44(7): 3366–3385. doi: 10.1109/TPAMI.2021.3057446.
|
[11] |
LI Zhizhong and HOIEM D. Learning without forgetting[J]. IEEE Transactions on Pattern Analysis and Machine Intelligence, 2018, 40(12): 2935–2947. doi: 10.1109/TPAMI.2017.2773081.
|
[12] |
RUSU A A, RABINOWITZ N C, DESJARDINS G, et al. Progressive neural networks[J]. arXiv: 1606.04671, 2016. doi: 10.48550/arXiv.1606.04671.
|
[13] |
RUDD E M, JAIN L P, SCHEIRER W J, et al. The extreme value machine[J]. IEEE Transactions on Pattern Analysis and Machine Intelligence, 2018, 40(3): 762–768. doi: 10.1109/TPAMI.2017.2707495.
|
[14] |
SHAO Junming, HUANG Feng, YANG Qinli, et al. Robust prototype-based learning on data streams[J]. IEEE Transactions on Knowledge and Data Engineering, 2018, 30(5): 978–991. doi: 10.1109/TKDE.2017.2772239.
|
[15] |
LI Bin, CUI Zongyong, CAO Zongjie, et al. Incremental learning based on anchored class centers for SAR automatic target recognition[J]. IEEE Transactions on Geoscience and Remote Sensing, 2022, 60: 5235313. doi: 10.1109/TGRS.2022.3208346.
|
[16] |
BORGWARDT K M, GRETTON A, RASCH M J, et al. Integrating structured biological data by kernel maximum mean discrepancy[J]. Bioinformatics, 2006, 22(14): e49–e57. doi: 10.1093/bioinformatics/btl242.
|
[17] |
王智睿, 康玉卓, 曾璇, 等. SAR-AIRcraft-1.0: 高分辨率SAR飞机检测识别数据集[J]. 雷达学报, 2023, 12(4): 906–922. doi: 10.12000/JR23043.
WANG Zhirui, KANG Yuzhuo, ZENG Xuan, et al. SAR-AIRcraft-1.0: High-resolution SAR aircraft detection and recognition dataset[J]. Journal of Radars, 2023, 12(4): 906–922. doi: 10.12000/JR23043.
|
[18] |
HUANG Lanqing, LIU Bin, LI Boying, et al. OpenSARShip: A dataset dedicated to Sentinel-1 ship interpretation[J]. IEEE Journal of Selected Topics in Applied Earth Observations and Remote Sensing, 2018, 11(1): 195–208. doi: 10.1109/JSTARS.2017.2755672.
|
[19] |
LI Bin, CUI Zongyong, SUN Yuxuan, et al. Density coverage-based exemplar selection for incremental SAR automatic target recognition[J]. IEEE Transactions on Geoscience and Remote Sensing, 2023, 61: 5211713. doi: 10.1109/TGRS.2023.3293509.
|
数据集 | 积极 | 中性 | 消极 |
Twitter-train | 1561 | 3127 | 1560 |
Twitter-test | 173 | 346 | 173 |
Laptop-train | 994 | 464 | 870 |
Laptop-test | 341 | 169 | 128 |
Restaurant-train | 2164 | 637 | 807 |
Restaurant-test | 728 | 196 | 196 |
实验环境 | 具体信息 |
操作系统 | Windows 10 教育版 |
CPU | Intel(R) Core(TM) i7-7700 CPU @ 3.60 GHz |
内存 | 16.0 GB |
显卡 | GTX 1080 |
显存 | 8.0 GB |
超参数 | 超参数值数量 |
词嵌入维度 | 300 |
隐藏状态向量维度 | 300 |
Batch size | 16 |
训练迭代次数epoch | 100 |
优化器Optimizer | Adam |
学习率Learning rate | 0.001 |
Dropout rate | 0.3 |
L2正则化系数 | 0.00001 |
模型 | Laptop | Restaurant | ||||||
准确率(Acc) | 宏平均F1 | 准确率(Acc) | 宏平均F1 | 准确率(Acc) | 宏平均F1 | |||
SVM | 63.40 | 63.30 | 70.49 | N/A | 80.16 | N/A | ||
LSTM | 69.56 | 67.70 | 69.28 | 63.09 | 78.13 | 67.47 | ||
MemNet | 71.48 | 69.90 | 70.64 | 65.17 | 79.61 | 69.64 | ||
IAN | 72.50 | 70.81 | 72.05 | 67.38 | 79.26 | 70.09 | ||
AOA | 72.30 | 70.20 | 72.62 | 67.52 | 79.97 | 70.42 | ||
AOA-MultiACIA | 72.40 | 69.40 | 75.27 | 70.24 | 82.59 | 72.13 | ||
ASGCN | 72.15 | 70.40 | 75.55 | 71.05 | 80.77 | 72.02 | ||
GATs | 73.12 | 71.25 | 74.61 | 70.51 | 80.63 | 70.41 | ||
IGATs | 75.29 | 73.40 | 76.02 | 72.05 | 82.32 | 73.99 |
模型 | 可训练参数数量 |
SVM | – |
LSTM | 0.72 |
MemNet | 0.36 |
IAN | 2.17 |
AOA | 2.10 |
ASGCN | 2.17 |
GATs | 1.81 |
IGATs | 1.81 |
模型 | Laptop | Restaurant | ||||||
准确率(Acc) | 宏平均F1 | 准确率(Acc) | 宏平均F1 | 准确率(Acc) | 宏平均F1 | |||
BiLSTM+IAtt | 74.13 | 72.86 | 75.08 | 70.82 | 81.25 | 72.14 | ||
BiLSTM+GAT+IAtt | 74.86 | 72.98 | 74.92 | 71.08 | 82.05 | 73.45 | ||
BiLSTM+PE+IAtt | 74.42 | 72.35 | 76.65 | 72.75 | 82.23 | 74.01 | ||
IGATs | 75.29 | 73.40 | 76.02 | 72.05 | 82.32 | 73.99 |
数据集 | 积极 | 中性 | 消极 |
Twitter-train | 1561 | 3127 | 1560 |
Twitter-test | 173 | 346 | 173 |
Laptop-train | 994 | 464 | 870 |
Laptop-test | 341 | 169 | 128 |
Restaurant-train | 2164 | 637 | 807 |
Restaurant-test | 728 | 196 | 196 |
实验环境 | 具体信息 |
操作系统 | Windows 10 教育版 |
CPU | Intel(R) Core(TM) i7-7700 CPU @ 3.60 GHz |
内存 | 16.0 GB |
显卡 | GTX 1080 |
显存 | 8.0 GB |
超参数 | 超参数值数量 |
词嵌入维度 | 300 |
隐藏状态向量维度 | 300 |
Batch size | 16 |
训练迭代次数epoch | 100 |
优化器Optimizer | Adam |
学习率Learning rate | 0.001 |
Dropout rate | 0.3 |
L2正则化系数 | 0.00001 |
模型 | Laptop | Restaurant | ||||||
准确率(Acc) | 宏平均F1 | 准确率(Acc) | 宏平均F1 | 准确率(Acc) | 宏平均F1 | |||
SVM | 63.40 | 63.30 | 70.49 | N/A | 80.16 | N/A | ||
LSTM | 69.56 | 67.70 | 69.28 | 63.09 | 78.13 | 67.47 | ||
MemNet | 71.48 | 69.90 | 70.64 | 65.17 | 79.61 | 69.64 | ||
IAN | 72.50 | 70.81 | 72.05 | 67.38 | 79.26 | 70.09 | ||
AOA | 72.30 | 70.20 | 72.62 | 67.52 | 79.97 | 70.42 | ||
AOA-MultiACIA | 72.40 | 69.40 | 75.27 | 70.24 | 82.59 | 72.13 | ||
ASGCN | 72.15 | 70.40 | 75.55 | 71.05 | 80.77 | 72.02 | ||
GATs | 73.12 | 71.25 | 74.61 | 70.51 | 80.63 | 70.41 | ||
IGATs | 75.29 | 73.40 | 76.02 | 72.05 | 82.32 | 73.99 |
模型 | 可训练参数数量 |
SVM | – |
LSTM | 0.72 |
MemNet | 0.36 |
IAN | 2.17 |
AOA | 2.10 |
ASGCN | 2.17 |
GATs | 1.81 |
IGATs | 1.81 |
模型 | Laptop | Restaurant | ||||||
准确率(Acc) | 宏平均F1 | 准确率(Acc) | 宏平均F1 | 准确率(Acc) | 宏平均F1 | |||
BiLSTM+IAtt | 74.13 | 72.86 | 75.08 | 70.82 | 81.25 | 72.14 | ||
BiLSTM+GAT+IAtt | 74.86 | 72.98 | 74.92 | 71.08 | 82.05 | 73.45 | ||
BiLSTM+PE+IAtt | 74.42 | 72.35 | 76.65 | 72.75 | 82.23 | 74.01 | ||
IGATs | 75.29 | 73.40 | 76.02 | 72.05 | 82.32 | 73.99 |